Na Zheng Xuemeng Song Qingying Niu Xue Dong Yibing Zhan Liqiang Nie
Collocation and Try-on Network: Whether an outfit is Compatible
Accepted to ACM MM 2021
Abstract
Whether an outfit is compatible? Using machine learning methods to assess an outfit’s compatibility, namely, fashion compatibility modeling (FCM), has recently become a popular yet challenging topic. However, current FCM studies still perform far from satisfactory, because they only consider the collocation compatibility modeling, while neglecting the natural human habits that peoplegenerally evaluate outfit comp-atibility from both the collocation (discrete assess) and the try-on (unified assess) perspectives. In light of the above analysis, we propose a Collocation and Try-On Network (CTO-Net) for FCM, combining both the collocation and try-on compatibilities. In particular, for the collo-cation perspective, we devise a disentangled graph learning scheme, where the collocation compatibility is disentangled into multiple fine-grained compatibilities between items; regarding the try-on perspective, we propose an integrated distillation learning scheme to unify all item information in the whole outfit to evaluate the compatibility based on the latent try-on representation. To further enhance the collocati-on and try-on compatibilities, we exploit the mutual learning strategy to obtain a more comprehensive judgment. Extensive experiments on the real-world dataset demonstrate that our CTO-Net significantly outperforms the state-of-the-art methods. In particular, compared to the competitive counter parts, our proposed CTO-Net significantly improves AUC accuracy from 83.2% to 87.8% and MRR from 15.4% to 21.8%. We will release oursource codes and trained models later.
Task
Figure 1: Illustration of the compatibility modeling fromboth collocation and try-on perspectives.
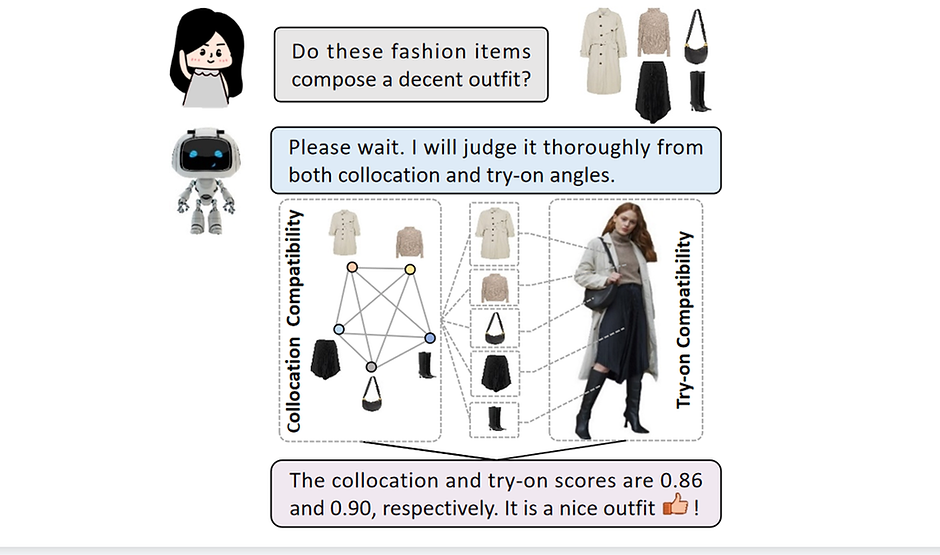
Framework
Figure 2: Illustration of the proposed CTO-Net, consisting of two core parts: CCM and TCM, responsible for mode-ling the fine-grained collocation compatibility and the uni-fied try-on compatibility, respectively. Finally, the mutual learning is employed to enc-ourage the knowledge sharing between them.
